
Abstract
The Lucas & Kanade (LK) algorithm is the method of choice for efficient dense image and object alignment. The approach is efficient as it attempts to model the connection between appearance and geometric displacement through a linear relationship that assumes independence across pixel coordinates. A drawback of the approach, however, is its generative nature. Specifically, its performance is tightly coupled with how well the linear model can synthesize appearance from geometric displacement, even though the alignment task itself is associated with the inverse problem. In this paper, we present a new approach, referred to as the Conditional LK algorithm, which: (i) directly learns linear models that predict geometric displacement as a function of appearance, and (ii) employs a novel strategy for ensuring that the generative pixel independence assumption can still be taken advantage of. We demonstrate that our approach exhibits superior performance to classical generative forms of the LK algorithm. Furthermore, we demonstrate its comparable performance to state-of-the-art methods such as the Supervised Descent Method with substantially less training examples, as well as the unique ability to "swap" geometric warp functions without having to retrain from scratch. Finally, from a theoretical perspective, our approach hints at possible redundancies that exist in current state-of-the-art methods for alignment that could be leveraged in vision systems of the future.
Videos
Code
The code is hosted on GitHub (MATLAB).
Publications
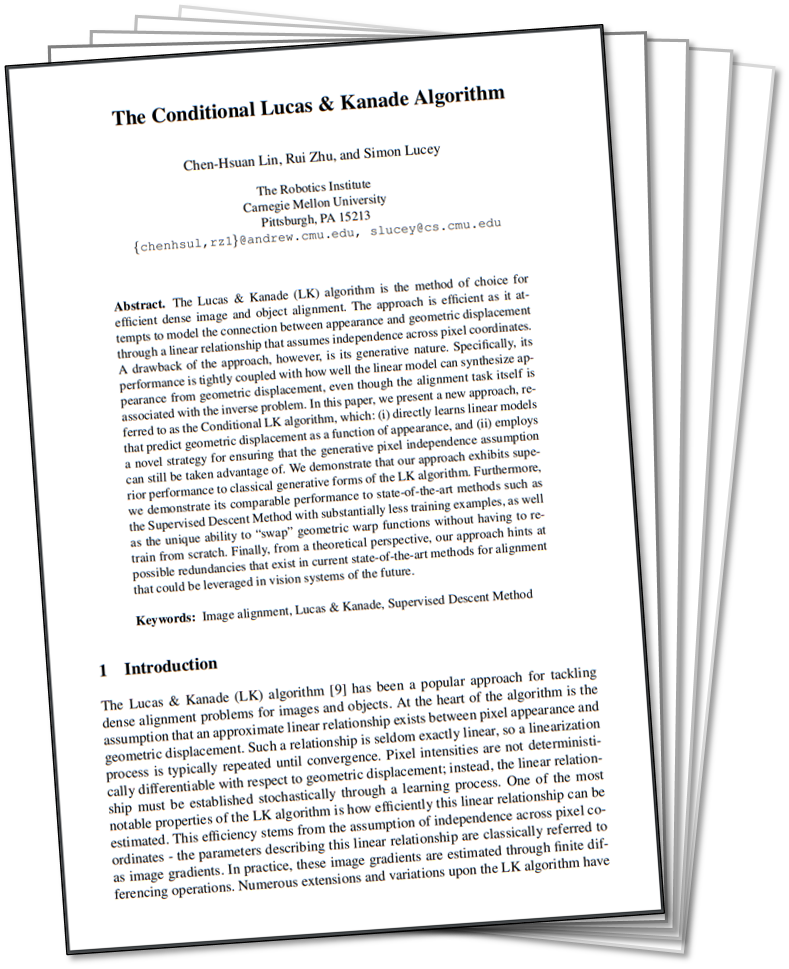
ECCV 2016 paper: https://arxiv.org/abs/1603.08597
BibTex:
@inproceedings{lin2016conditional,
title={The Conditional Lucas \& Kanade Algorithm},
author={Lin, Chen-Hsuan and Zhu, Rui and Lucey, Simon},
booktitle={European Conference on Computer Vision (ECCV)},
pages={793--808},
year={2016},
organization={Springer International Publishing}
}
title={The Conditional Lucas \& Kanade Algorithm},
author={Lin, Chen-Hsuan and Zhu, Rui and Lucey, Simon},
booktitle={European Conference on Computer Vision (ECCV)},
pages={793--808},
year={2016},
organization={Springer International Publishing}
}